Amongst all the other things that keep us up at night, one of them has to be the effect that AI has over us and how it’s becoming a significant part of our decision-making, influencing everything from loan approvals to job recruitment.
The global AI market, expected to reach $1.8 trillion by 2030, offers ample opportunities but also raises ethical questions.
Generative AI (GenAI) is a type of AI that creates new content, like text, images, music, or videos, by learning from large amounts of existing data.
While this makes GenAI very useful in many fields, it also comes with challenges like creating unfair or biased content, sharing private information, or being a black box without any way of knowing how it ended up generating something"
In this blog, we will see how to approach these challenges responsibly so that GenAI is beneficial and inclusive for everyone.

Privacy
Generative AI uses huge amounts of data to create customized results, like suggestions or content.
But using so much data brings important questions about keeping it safe, getting proper permission, and protecting it from misuse.
Data security: As of 2023, 83% of companies reported experiencing data breaches, highlighting the need for stronger protections.
Sensitive information, like health records and shopping histories, needs to be protected.
Companies using generative AI can take steps to keep data safe and build trust. This includes locking sensitive data with strong encryption, regularly checking for security risks, and limiting access to only those who need it.
Consent and transparency: Research shows that 72% of users are unaware of how their data is collected or used.
Bias
AI systems learn from past data, which often reflects societal biases. If these biases aren't dealt with, they can result in unfair decisions in areas like hiring, healthcare, law enforcement etc.
Training data bias: A 2019 study found that AI recruiting tools favored male candidates over female candidates because they were trained on biased data. Regular checks and using a wide range of data can help reduce these problems.
Representation bias: Facial recognition systems, for instance, have an error rate of 34% for darker-skinned women compared to just 1% for lighter-skinned men.
This gap highlights the importance of using fair and diverse data for training can help reduce these gaps and make AI systems more equitable.
Transparency
The challenge with GenAI is that it works like a “black box” – people don’t always know how it makes decisions. This lack of clarity can make it hard to trust the results or hold anyone responsible when something goes wrong.
Trust and clarity: A 2022 survey revealed that 60% of consumers are hesitant to use AI-driven systems because they don’t understand how decisions are made.
AI systems need to clearly explain their decisions, like why they approved a loan or suggested medical treatment, in a way that’s easy for anyone to understand.
Ethical oversight: In important areas like healthcare and criminal justice, being clear is a must. When decisions are easy to understand, it’s simpler to spot mistakes or unfairness and hold organizations responsible for them.
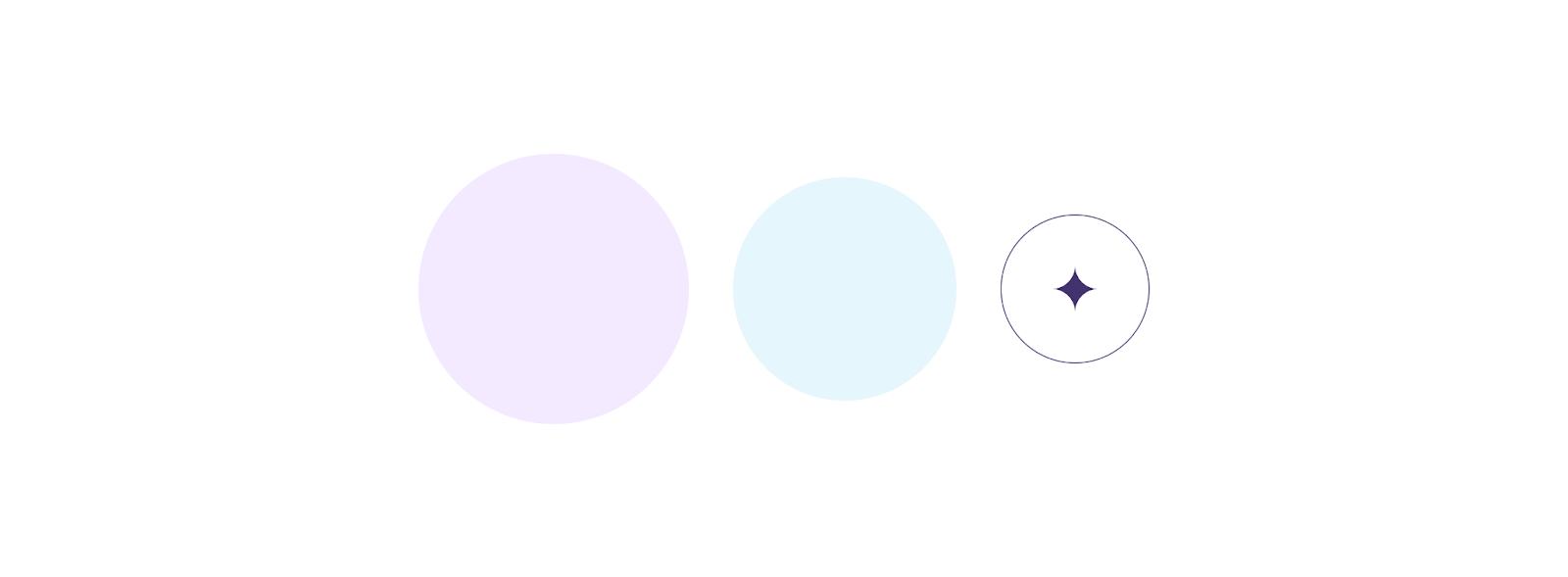
Navigating ethical challenges with practical solutions
To build fair and trustworthy AI, businesses need to focus on three key areas:
Protecting privacy
People are concerned about how their personal information is used. To build trust, businesses need to handle data carefully.
Keep data anonymous: Remove personal details from data to protect people’s identities while still making the information useful.
For example, streaming services can analyze viewing habits without revealing personal accounts.
Use privacy techniques: Differential privacy is a way to protect individual data by adding random changes, or noise, to it. This lets companies find trends without revealing personal details.
Apple uses this approach to improve features like QuickType suggestions and emoji insights while keeping user data private.
Follow data laws: Laws like GDPR in Europe or CCPA in California require businesses to safeguard personal data.
Meeting these standards not only protects people but also builds customer trust.
Reducing bias
AI systems often reflect the biases in the data they’re trained on, which can lead to unfair outcomes.
Use Diverse data: Train AI on data that includes people of different backgrounds, ages, and experiences.
For example, adding accents and regional dialects improves speech recognition for all users.
Check for fairness regularly: Businesses should test their systems frequently to catch and fix unfair outcomes.
Tools like Google’s What-If Tool make it easier to spot problems.
Build inclusive teams: Involve people from different backgrounds in AI development. A diverse team can help identify and solve bias issues early on.
Improving transparency
When people don’t understand how AI works, they’re less likely to trust it. A study by Deloitte found that 62% of consumers want AI systems to explain their decisions clearly.
Make AI explainable: Build systems that show how decisions are made in simple terms.
For example, some AI tools include features that let users see why certain outcomes were chosen.
Open up where possible: Share parts of your AI systems, like algorithms or training data, with trusted experts.
This adds credibility and allows others to confirm the system is fair.
Help people understand: Offer clear guides or examples that show users how your AI works and how their data is used. This makes them feel more in control.
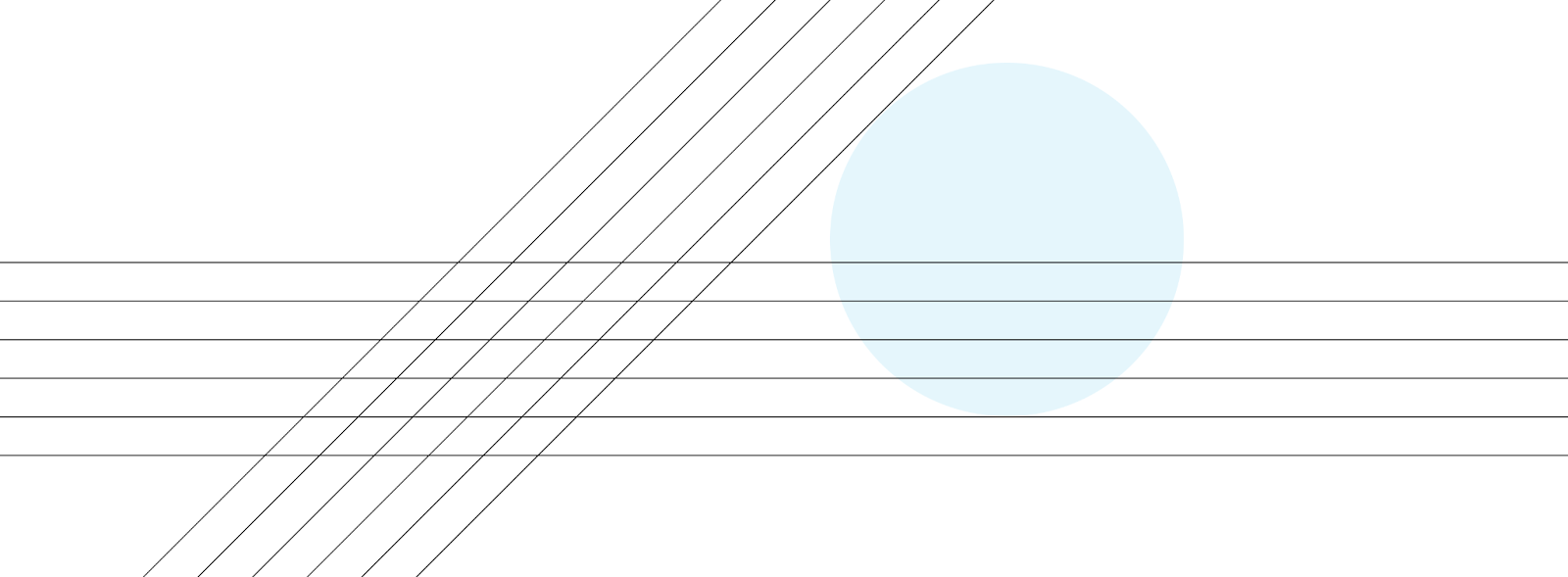
The future of ethical GenAI
Generative AI is already reshaping industries by tackling complex challenges, creating new opportunities, and delivering meaningful value across sectors like healthcare, finance, energy, and the creative economy.
In healthcare, AI-driven tools already analyze medical images with up to 90% accuracy, improving diagnostics and treatment outcomes.
Financial systems use AI to reduce fraud, a global issue costing businesses $5.38 trillion in 2023, while in energy, AI is accelerating breakthroughs in sustainable technologies like next-generation batteries.
The global generative AI market is projected to grow from $10.63 billion in 2022 to $200.73 billion by 2032, with applications spanning fraud detection, operational efficiency, and creative content.
Yet, for GenAI to truly serve its purpose, we must confront the ethical challenges it presents.
Safeguarding privacy ensures trust in systems that handle sensitive data. Tackling bias creates fairness, ensuring AI-driven decisions don't reinforce harmful stereotypes.
Transparency builds accountability, helping users and businesses understand and rely on AI systems with confidence.
Companies that focus on privacy, fairness, and transparency, won’t just lead their industries; they will set the standard for technology that supports humanity.