From assisting with surgeries and pharmaceutical development to improving patient communication and disease tracking, Artificial Intelligence is shaping how we address public health’s toughest challenges all while transforming care delivery on a global scale.
During critical moments like the COVID-19 pandemic, AI demonstrated its ability to forecast outbreaks, optimize vaccine distribution, and combat misinformation.
Beyond crisis scenarios, AI continues to revolutionize public health by analyzing vast datasets, identifying disease risks early, and guiding resource allocation to maximize impact.
However, with great potential comes responsibility. Public health systems must navigate through significant challenges to fully realize the benefits of AI.
This blog explores how AI is advancing personalized care, addressing systemic challenges, and paving the way for smarter, more equitable public health solutions.
How it all started
The journey of AI in public health spans over five decades, marked by significant technological leaps.
It began in the 1970s with systems like MYCIN, which used rule-based algorithms to help doctors with diagnoses and treatments. While innovative, these early systems were limited in handling large data or adapting to new situations.
By the 1990s, advances in computing enabled AI to process larger datasets, paving the way for systems like the Early Warning and Response System to detect disease outbreaks.
In the 2000s, AI used machine learning for public health trends, with solutions like Google Flu Trends estimating flu activity via search data.
In 2019, BlueDot demonstrated AI’s power by detecting COVID-19 nine days before official warnings.
Today, AI maps disease hotspots, optimizes vaccine distribution, and predicts risks, supported by organizations like the WHO and CDC.
Despite progress, challenges like data privacy and equitable access remain, highlighting the need for careful integration to realize AI's transformative potential in public health.
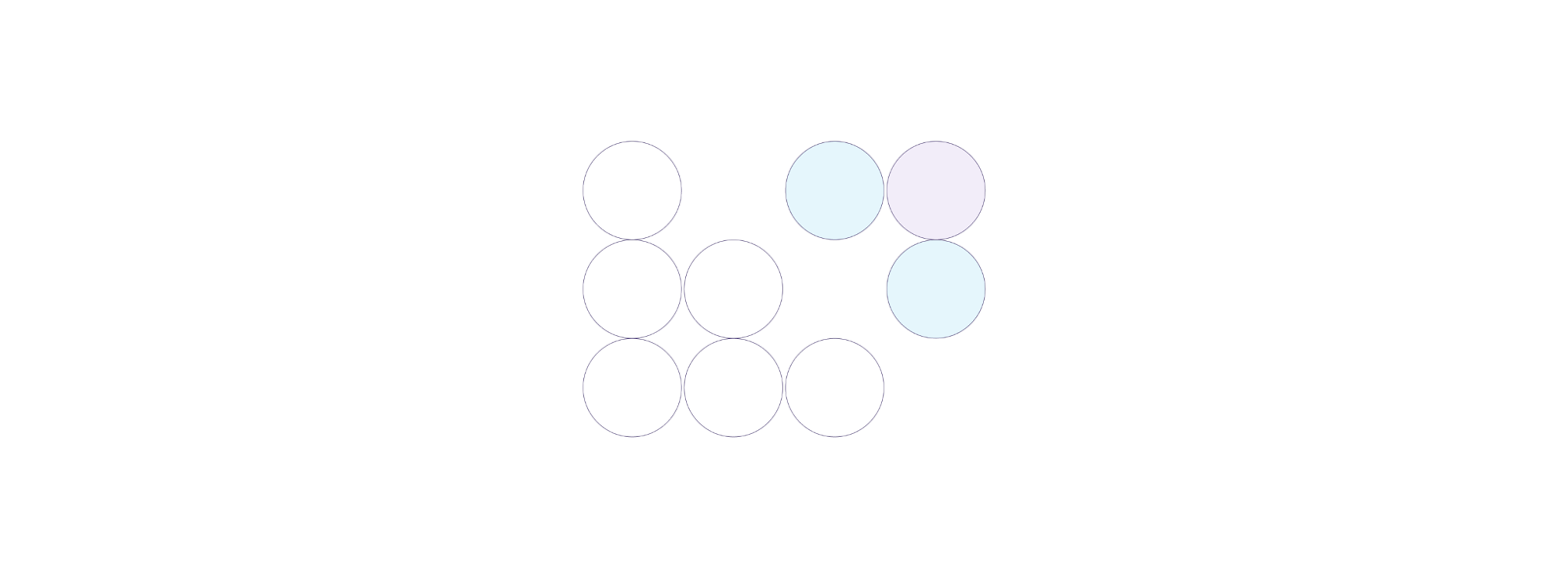
The hurdles we face
Privacy concerns
Health data is highly sensitive, and its use in AI raises substantial privacy risks.
Unauthorized access or breaches can lead to misuse of personal information, as seen in debates over tech companies accessing NHS archives for AI research.
Access disparities
AI’s benefits often favor regions with advanced infrastructure, leaving low-income areas behind.
Many communities lack the technology and connectivity to leverage AI tools, further widening global health disparities.
Algorithmic bias
AI models are only as good as the data they are trained on. If that data lacks diversity, the results can perpetuate biases, leading to unequal healthcare outcomes.
For example, models trained predominantly on Western datasets may fail to perform accurately for other populations.
Ethics and regulation
Clear guidelines for the ethical use of AI in healthcare are still in development.
The FDA’s efforts to create tailored regulations for AI-based medical devices highlight the need for oversight.
Transparency in AI models
Many AI systems operate as “black boxes,” with decision-making processes that are hard to interpret. This lack of transparency can lead to mistrust among healthcare providers and patients.
Developing interpretable AI systems is crucial for their broader acceptance
Interoperability
AI solutions often struggle to integrate with existing healthcare systems, particularly in regions with diverse infrastructure.
Creating solutions that are compatible across systems is critical for effective implementation.
Cost and scalability
AI technologies can be expensive to develop and implement, particularly in resource-constrained settings.
Finding cost-effective ways to scale AI solutions remains a major hurdle
Cultural and linguistic barriers
AI tools must account for cultural and linguistic differences to be effective globally.
Without proper adaptation, models trained on one region’s data may not perform well elsewhere .
Workforce readiness
Healthcare professionals often lack training in AI technologies, limiting their ability to use these solutions effectively.
Including AI education in medical training programs is crucial for successful adoption.
Long-term data reliability
AI relies on historical data, which must be continuously updated to remain accurate.

How AI is transforming public health solutions
AI offers transformative solutions in public health, addressing the issues in early detection, personalized care, resource management, and health communication.
Here are the key areas where AI is making a difference
Early disease detection and surveillance
AI Platforms like ProMED analyze large datasets like medical records and environmental data to detect outbreaks early.
Predictive models assess disease spread patterns, aiding in timely interventions. The World Health Organization’s Epidemic Intelligence from Open Sources (EIOS) supports global disease surveillance using AI.
Personalized healthcare
AI tailors treatments by analyzing patient-specific data. For instance, mental health apps like Woebot use AI to offer real-time support for anxiety and depression.
AI in cancer care helps doctors design personalized treatment strategies. Research, such as a study published in Nature Medicine, shows AI’s ability to detect breast cancer more accurately than radiologists.
Resource optimization
Predictive models improve resource allocation during emergencies. AI frameworks like DeepMind’s Streams App optimize hospital bed usage and patient prioritization.
For example, Operation Warp Speed utilized AI for supply chain optimization
Improving access to reliable information
AI chatbots, like Ada Health, provide accurate health guidance to patients and caregivers, reducing misinformation.
Post-discharge monitoring digital healthcare platform, such as Babylon Health, helps track patient recovery and ensure continuity of care.
Predictive analytics for long-term planning
AI systems analyze environmental and social data to predict long-term health trends.
For example, IHME’s Global Burden of Disease project leverages AI to forecast health challenges like chronic diseases.
Predictive analytics also support planning for emerging threats like antimicrobial resistance (CDC on AMR).
Supporting health equity
AI addresses health disparities by identifying underserved communities.
Projects like Data for Good at Meta use AI to map healthcare access and needs in vulnerable populations.
Vaccine allocation models, such as those developed by UNICEF, use AI to prioritize high-risk groups.
Medical research and drug development
AI accelerates drug discovery, reducing time and costs. Platforms like Insilico Medicine use AI to predict viable drug candidates.
Machine learning models, such as those developed by IBM Watson Health, assist in optimizing clinical trials and participant selection.
By leveraging these AI solutions, public health systems can enhance efficiency, equity, and outcomes on a global scale.
Looking forward
The stakes are enormous, but so is the opportunity with AI to transform how care is delivered to the most vulnerable population.
With the right focus on equity, collaboration, and responsible use, AI can bridge gaps in healthcare access, empower decision-makers, and make public health systems smarter.
For instance, AI algorithms trained on vast datasets can outperform experienced doctors in detecting conditions like skin cancer and breast cancer risk.
AI models like Sybil are leading the way in predicting health outcomes using current and future data trends, paving the path for personalized, forward-looking care.
Studies at institutions like MIT show that hybrid human-AI models excel in scenarios such as identifying cardiomegaly in chest X-rays.
Beyond diagnostics, AI applications are making global health systems more efficient, from allocating resources to forecasting disease outbreaks.
However, the road ahead requires a strong focus on equity and community-driven solutions. AI should benefit everyone, not just those with privileged access.
By building partnerships and adhering to principles of fairness and accessibility, AI can help create a healthier, more equitable world.